Mathematics Colloquium
Upcoming Colloquium
Mathematical Investigations of Kinetoplast DNA
Dr. Alex Klotz, CSU Long Beach
April 18, 2025
12:00pm-1:00pm in FO3-200A and via Zoom
Join 4/18 Zoom
Meeting ID: 881 9106 9435
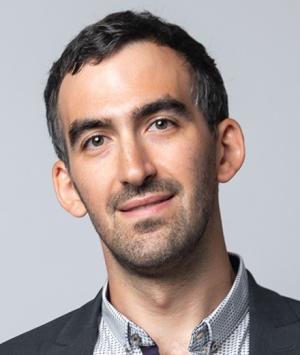
Abstract
Kinetoplast DNA, often described as molecular chainmail, is found in the mitochondria of trypanosome parasites and consists of thousands of topologically interlocked circular molecules. In addition to its biological role in gene editing, it has been explored recently as a model system for materials science, due to its unique topological connectivity and its two-dimensional structure. In this talk, I will discuss some mathematical investigations that have emerged out of materials-based research of kinetoplast DNA, including the relationship between the link topology of the network and the Gaussian curvature of chainmail membranes, as well as methods to detect Borromean linking within densely linked networks.
Biosketch
Alex Klotz has been a professor in the Department of Physics and Astronomy at CSULB since 2019. Prior to that, he received his Ph.D. From McGill University and was a postdoctoral fellow at MIT. His research, both experimental and theoretical, focuses on topologically complex biomolecules and related systems.
About the Colloquium
The Mathematics Colloquium is a unique opportunity for students to learn about new developments in mathematics and what mathematics and statisticians do after they graduate. Hosted by the Department of Mathematics and Statistics at California State University, Long Beach, the weekly meetings invite guests from universities, research laboratories, and industry to present and discuss current topics in mathematics. All students are encouraged to attend.
Schedule
The Spring 2025 will be posted as it becomes available.
Date | Title | Speaker and Affiliation |
---|---|---|
April 18, 2025 | Mathematical Investigations of Kinetoplast DNA | Dr. Alex Klotz, CSU Long Beach |
April 25, 2025 | TBD | Jesse Wolfson, UC Irvine |
May 2, 2025 | Exploring practice-based experiences in mathematics teacher education: Rehearsals, scenarios, and simulations | Erin Barno, Educational Testing Service; Liza Bondurant, Mississippi State; Madelyn Colonnese, University of North Carolina - Charlotte; Heather Howel, Eastern Carolina University; Carrie Lee, independent; Bima Sapkota, University of Texas, Rio Grande Valley |
Previous Colloquia
The following are colloquia from Spring 2025. The Mathematics Colloquium Archive has the colloquia from previous semesters.
Causal inference and machine learning in mobile health --- modeling time-varying effects using longitudinal functional data
Dr. Tianchen Qian, UC Irvine
April 4, 2025
Abstract
To optimize mobile health interventions and advance domain knowledge on intervention design, it is critical to understand how the intervention effect varies over time and with contextual information. This study aims to assess how a push notification suggesting physical activity influences individuals' step counts using data from the HeartSteps micro-randomized trial (MRT). The statistical challenges include the time-varying treatments and the longitudinal functional step count measurements. We propose the first semiparametric causal excursion effect model with varying coefficients to model the time-varying effects within a decision point and across decision points in an MRT. The proposed model incorporates double time indices to accommodate the longitudinal functional outcome, enabling the assessment of time-varying effect moderation by contextual variables. We propose a two-stage causal effect estimator that is robust against a misspecified high-dimensional outcome regression nuisance model. We establish asymptotic theory and conduct simulation studies to validate the proposed estimator. Our analysis provides new insights into individuals' change in response profiles (such as how soon a response occurs) due to the activity suggestions, how such changes differ by the type of suggestions received, and how such changes depend on other contextual information such as being recently sedentary and the day being a weekday.
Biosketch
Tianchen Qian is an Assistant Professor of Statistics at the University of California, Irvine. His research interests include causal inference, experimental design, machine learning, semiparametric efficiency theory, and longitudinal data analysis. He applies these methods to develop personalized digital interventions to improve health and well-being at scale. In addition, he conducts collaborative research on Alzheimer’s disease and related dementia. Tianchen earned his PhD in Biostatistics from Johns Hopkins University and completed a postdoctoral fellowship in Statistics at Harvard University before joining UCI.
Some Theory and Practice of AI and Data Science in Research and in the Classroom
Dr. Steven Heilman, USC
March 21, 2025
Abstract
We will begin with an overview of some data science methods such as singular value decomposition, clustering and deep learning, as applied to some examples from an undergraduate course in Data Science. We will then discuss some recent use of large language models (LLMs) for solving mathematical problems. Along the way, we will discuss the deficiencies of the optimization method used to train LLMs, and some theory on aggregating outputs of LLMs using plurality voting.
Biosketch
Steven Heilman is an Assistant Professor RTPC of Mathematics at University of Southern California working in probability, theoretical computer science, and data science. Steven held previous positions at Notre Dame and UCLA, receiving a Ph.D. in Mathematics at Courant Institute, NYU and a BA in Mathematics at Cornell University.
Combinatorics to Knot Theory (and Back Again)
Dr. Nicolle Gonzalez, UC Berkeley
March 7, 2025
Abstract
Catalan numbers are among the most ubiquitous objects in mathematics, arising naturally in combinatorics, representation theory, geometry, and many other areas. Although there are various polynomial generalizations of these numbers, particularly fruitful are the so-called (q,t)-Catalan polynomials. Among many other things, these polynomials provide a direct link between combinatorial objects, such as Dyck paths and parking functions, and the Khovanov-Rozansky homology (a particular homological link invariant) of so-called torus knots. In this talk, I will explain some of the fascinating connections—both known and conjectural—between various Catalan objects and knot theory. I will also present new families of Catalan polynomials, constructed by my collaborators and me, that generalize previous formulations and provide new insights into the Khovanov-Rozansky homology for the larger family of Coxeter knots.
Biosketch
Nicolle González is a Venezuelan mathematician working at UC Berkeley as a Morrey Visiting Assistant Professor working in the intersection of representation theory and algebraic combinatorics, motivated by questions in low dimensional topology and categorification. She got her PhD at the University of Southern California and was a Presidential Postdoctoral Fellow at UCLA and a Berlekamp Fellow at MSRI.
Using Novel Representations of Practice for Teachers' Professional Noticing of Mathematics: Research & Practice
Dr. Karl Kosko, Kent State University
February 28, 2025
Abstract
Teacher noticing is an important area of educational research that focuses on teachers' ability to attend to and interpret key pedagogical events in a teaching context. Although it is most authentic when teachers are in the classroom, both research and professional development have used various representations of practice (video, scanned student work, etc.) for practicality. This presentation presents results and findings from both research and practice on the use of novel, immersive representations such as virtual and extended reality, and the use of eye-tracking enabled videos. Use of such representations significantly increase teachers' feeling of presence in the recordings and increase what and how they notice, but present new challenges both for research and practice.
Biosketch
Karl Kosko is a former classroom teacher and current Professor in Mathematics Education at Kent State University. His research interest focuses on how mathematical meaning is conveyed, including studies of mathematical argument & discourse, multiplicative reasoning, and using representations of practice in elementary mathematics teacher education.
Strict Comparison in C*-algebras
Gregory Patchell, Ph.D. candidate, UC San Diego
February 14, 2025
Abstract
One of the most fundamental ways to compare matrices is via their rank. For two matrices X and Y, rank(X) is less than or equal to rank(Y) if and only if there are matrices S and T such that X = SYT. C*-algebras are infinite-dimensional analogs of matrix algebras. The rank can be generalized to C*-algebras using dimension functions and the latter algebraic condition can be generalized to a condition known as Cuntz subequivalence. C*-algebras for which the dimension functions recover Cuntz subequivalence are said to have strict comparison. Strict comparison is known to have applications to the classification of C*-algebras, including the influential Toms-Winter conjecture. In 1998 Dykema-Rørdam showed that infinite reduced free products have strict comparison, but even for the free group on two generators strict comparison of the reduced group C*-algebra was a long-standing open problem. In our work (joint with Tattwamasi Amrutam, David Gao, and Srivatsav Kunnawalkam Elayavalli) we show that the reduced group C*-algebra of the free group on two generators has strict comparison. Our methods are very general and lead to proving strict comparison for all acylindrically hyperbolic groups with rapid decay.
Biosketch
Gregory Patchell is a PhD Candidate at UC San Diego and he will be a Postdoctoral Research Associate at Oxford University starting this fall. He grew up in Canada and attended the University of Waterloo for his undergraduate. His research is in operator algebras, especially when there are connections with analytic and geometric properties of groups.
Topological Deep Learning in Computer-Aided Drug Discovery and Beyond
Dr. Yuzhou Chen, UC Riverside
February 14, 2025
Abstract
In computer-aided drug discovery (CADD), virtual screening (VS) is used for identifying the drug candidates that are most likely to bind to a molecular target in a large library of compounds. Most VS methods to date have focused on using canonical compound representations or generating alternative fingerprints of the compounds by training progressively more complex variational autoencoders (VAEs) and graph neural networks (GNNs). Although VAEs and GNNs led to significant improvements in VS performance, these methods suffer from reduced performance when scaling to large virtual compound datasets. The performance of these methods has shown only incremental improvements in the past few years. To address this problem, we developed a novel method using multiparameter persistence (MP) homology that produces topological fingerprints of the compounds as multidimensional vectors. We further establish theoretical guarantees for the stability properties of our proposed MP signatures, and demonstrate that our models, enhanced by the MP signatures, outperform state-of-the-art methods on benchmark datasets by a wide and highly statistically significant margin.
Biosketch
Dr. Yuzhou Chen is an Assistant Professor in the Department of Statistics at University of California, Riverside. He is also an Adjunct Assistant Professor in the Department of Computer and Information Sciences at Temple University and a Visiting Research Collaborator in the Department of Electrical and Computer Engineering at Princeton University. Before joining Temple, he worked as a postdoctoral scholar in the Department of Electrical and Computer Engineering at Princeton University. He received his Ph.D. in Statistics at Southern Methodist University in 2021. His research focuses on geometric machine learning, topological data analysis, knowledge discovery in graphs and spatio-temporal data, with applications to biosurveillance, energy systems, intelligent transportation, cryptocurrency, and environmental sciences. His research has appeared in the top machine learning and data mining conferences and journals, including ICML, ICLR, NeurIPS, AAAI, PNAS, etc. He won 2022 and 2021 Best Paper Awards of the Section for SDNS of ASA and the 2021 Chateaubriand Fellowship from the Embassy of France in the United States.
Innovative Approaches to Mathematics Assessment Development for Linguistically Diverse Students
Dr. Melissa Gallagher, US Math Recovery Council
January 31, 2025
Abstract
Traditional assessments that are used to determine mathematics interventions for students have high language demands (Trakulphadetkrai et al., 2020), focus on deficits (Hunt et al., 2019; Myers, 2022; Rodríguez et al., 2022), and present learning in a linear progression, despite evidence that learning trajectories (LTs) are nonlinear (Confrey, 2018; Confrey & Maloney, 2012). The available evidence suggests that multilingual learners (MLs) are over-represented in pull out interventions and particularly impacted by the limitations of high language demand assessments. This presentation will describe an innovative measure development project aimed at overcoming the challenges of traditional assessments.
Biosketch
Melissa A. Gallagher, Ph.D., is the senior research and evaluation specialist at the US Math Recovery Council. Her research focuses on the knowledge, beliefs, and practices that teachers need to support multilingual students in the mathematics classroom. She enjoys learning about new research methods and employing them in novel ways to answer research questions.
The Mathematics Colloquium Archive has the Colloquia from previous semesters.
Colloquium Committee
For Spring 2025:
- Dr. Pavneet Kaur Bharaj
- Dr. Seungjoon Lee
- Dr. Rolando de Santiago